By T. Mertzimekis
This is not an original blog entry of mine, in the sense that I am just reposting a very interesting article on how two, seemingly different, disciplines stay under the same roof. The just published article on Physical Review Accelerators and Beams (see https://doi.org/10.1103/PhysRevAccelBeams.26.024801) deals with forecasting methods and particle accelerators. If you are interested on how STEM comes along with time series, this is a nice overview you can start with. Go ahead and stay tuned for more posts in that direction in the near future.
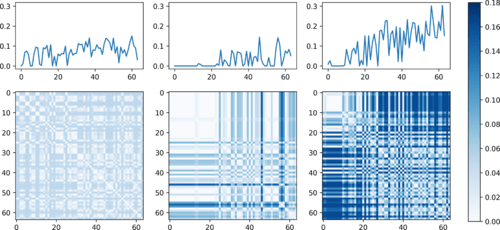
Get a glimpse from the abstract:
Particle accelerators are complex facilities that produce large amounts of structured data and have clear optimization goals as well as precisely defined control requirements. As such they are naturally amenable to data-driven research methodologies. The data from sensors and monitors inside the accelerator form multivariate time series. With fast preemptive approaches being highly preferred in accelerator control and diagnostics, the application of data-driven time series forecasting methods is particularly promising. This review formulates the time series forecasting problem and summarizes existing models with applications in various scientific areas. Several current and future attempts in the field of particle accelerators are introduced. The application of time series forecasting to particle accelerators has shown encouraging results and promise for broader use, and existing problems such as data consistency and compatibility have started to be addressed.